AI in credit risk evaluation: a game changer
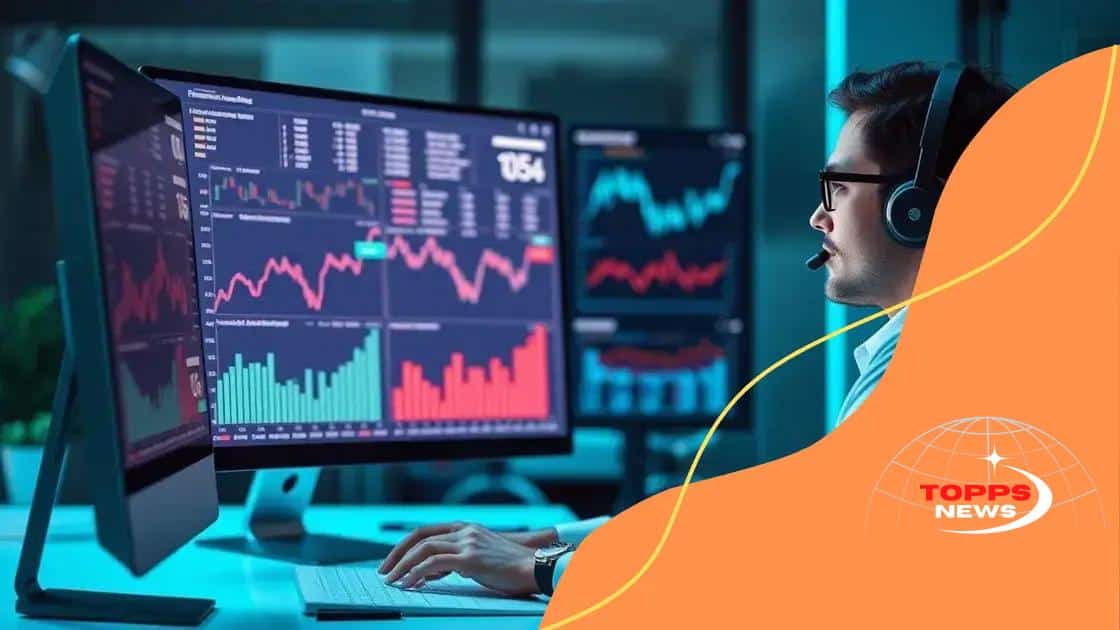
AI in credit risk evaluation enhances accuracy and efficiency by analyzing vast data, incorporating alternative information, and reducing biases, ultimately leading to more informed lending decisions.
AI in credit risk evaluation is transforming the financial landscape. By leveraging advanced algorithms, financial institutions can assess risks more accurately than ever. Have you ever wondered how these technologies are shaping lending practices today?
Understanding credit risk evaluation
Understanding credit risk evaluation is essential for financial institutions. This involves assessing the likelihood of a borrower defaulting on a loan. It seems complex, but with the right tools, it can be simplified significantly.
The Basics of Credit Risk Evaluation
At its core, credit risk evaluation determines how likely a borrower is to repay their debt. This process helps lenders make informed decisions about extending credit. Factors such as the borrower’s credit history, income level, and existing debts are typically analyzed.
Key Factors Influencing Credit Risk
- Credit Score: A numerical expression based on a level analysis of a borrower’s credit files.
- Debt-to-Income Ratio: A measure of a borrower’s monthly debt payments in relation to their monthly income.
- Employment History: A record of the borrower’s job stability and income consistency.
- Payment History: An account of past payments, including any missed or late payments.
Understanding these factors helps lenders not only determine risk but also aid in decision-making. Furthermore, evaluating credit risk accurately helps minimize potential losses. Lenders utilize various models, including statistical and algorithmic approaches.
Modern Approaches to Evaluation
Many institutions now leverage AI technologies for a more precise evaluation process. These technologies analyze vast amounts of data quickly, extracting useful insights that traditional methods may overlook. By continuously learning from new data, AI systems can improve their assessments over time.
Additionally, incorporating economic indicators can provide deeper insights into potential risks. By combining qualitative and quantitative data, institutions can develop a comprehensive understanding of risk involved in lending practices.
In conclusion, the journey of credit risk evaluation is evolving. As technology advances, so does the ability to assess risk with greater accuracy and efficiency. This safeguards both lenders and borrowers in the financial landscape.
How AI improves accuracy in assessments
How AI improves accuracy in assessments is a vital topic in financial technology. Utilizing AI can significantly enhance the precision of credit risk evaluations, making decisions more reliable.
Enhanced Data Analysis
AI systems can analyze massive amounts of data more quickly and accurately than humans. By processing historical data, AI identifies patterns that may go unnoticed. This helps institutions to make better predictions about a borrower’s risk.
Predictive Modeling Techniques
With advanced predictive modeling, AI algorithms can assess future risks based on existing data. These models can consider various factors, adapting as new information becomes available. As a result, financial decisions are less likely to be based on outdated information.
- Machine Learning: Machine learning algorithms improve over time, becoming more accurate with each data point.
- Natural Language Processing: AI can analyze written content such as applicants’ financial statements, extracting relevant insights.
- Real-Time Data Integration: AI can pull information from multiple sources in real-time, providing a comprehensive view of the borrower.
Using AI technology not only enhances accuracy but also speeds up the evaluation process. Traditional methods can be time-consuming, whereas AI processes assessments in a fraction of the time. This efficiency allows lenders to respond to borrowers more swiftly.
Additionally, AI reduces human error in evaluations. Biases that may affect manual assessments are minimized, leading to fairer lending practices. As AI continues to evolve, its role in improving assessment accuracy will only grow.
The integration of AI in financial assessments has proven beneficial across the board. Institutions that harness this technology can offer better services and make more informed decisions, ultimately leading to increased trust among borrowers.
Key benefits of AI in credit risk management
Key benefits of AI in credit risk management play a significant role in transforming how financial institutions operate. By integrating AI, companies can gain numerous advantages that streamline operations and enhance decision-making.
Improved Efficiency
AI technologies can process data at remarkable speeds. This rapid analysis allows lenders to evaluate credit applications quickly, reducing wait times for borrowers. Consequently, banks can increase their efficiency while serving more customers.
Enhanced Risk Assessment
With AI, risk assessment is more precise. Algorithms analyze vast datasets, identifying patterns and trends that human analysts might miss. This results in a clearer understanding of a borrower’s risk profile.
- Data-Driven Insights: AI can provide deeper insights based on historical data, improving predictions about a borrower’s behavior.
- Real-Time Monitoring: Institutions can continuously track borrowers’ behavior, allowing for proactive risk management.
- Adaptive Learning: AI systems can learn and adapt to new data, refining their assessments over time.
Furthermore, AI can mitigate human biases in the evaluation process. By relying on data, lenders can make more objective decisions, which leads to fairer outcomes. This transparency can build trust between lenders and borrowers, fostering long-term relationships.
The ability for AI systems to eliminate repetitive tasks also frees staff to focus on more complex decision-making. This shift not only improves workplace efficiency but also enhances job satisfaction.
Finally, leveraging AI can lead to significant cost savings for financial institutions. By improving accuracy and efficiency, companies can reduce their operational costs, making resources available for other strategic initiatives.
Challenges when implementing AI solutions
Challenges when implementing AI solutions in credit risk management can be significant. While AI offers many benefits, some hurdles may hinder its successful adoption.
Data Quality Issues
One major challenge is ensuring the quality of data used for AI training. Poor-quality data can lead to inaccurate predictions and assessments. Financial institutions must invest in data cleaning and management to ensure that they use reliable information.
Integration with Existing Systems
Another challenge is integrating new AI systems with existing technology. Many banks operate with legacy systems that may not easily connect with modern AI platforms. This integration requires careful planning and resources.
- Cost of Implementation: Deploying AI solutions can be expensive as organizations invest in both technology and training.
- Staff Training: Employees need proper training to work effectively with AI tools, which can take time and effort.
- Ethical Considerations: Using AI raises concerns around privacy and bias. Organizations must ensure their AI systems are fair and transparent.
Moreover, the fast-paced evolution of AI technology means businesses must stay updated with current trends. This requirement can create additional pressure as institutions strive to maintain a competitive edge.
There’s also the challenge of public perception. Borrowers may have concerns about relying on AI for credit decisions. To gain trust, institutions need to communicate the benefits and reliability of their AI systems effectively.
Despite these challenges, many organizations are finding ways to overcome obstacles and reap the benefits of AI in credit risk management. By addressing these issues head-on, institutions can pave the way for a more efficient, data-driven future.
Future trends in AI and credit risk evaluation
Future trends in AI and credit risk evaluation are poised to reshape the financial industry. As technology advances, so do the methodologies for assessing credit risk.
Increased Use of Machine Learning
One major trend is the continued rise of machine learning algorithms. These algorithms improve efficiency by learning from new data. They can adapt quickly to changes in borrower behavior, making credit evaluations more accurate over time.
Integration of Alternative Data
Another exciting direction is the integration of alternative data sources. Traditional credit scores do not always reflect a borrower’s true risk. By incorporating data such as social media activity, payment history on utility bills, and transaction data, lenders can gain a fuller picture of the borrower’s creditworthiness.
- Enhanced Personalization: Personalized credit offers may emerge as institutions better understand individual risks.
- Real-Time Decision Making: AI will facilitate instantaneous processing of applications, resulting in quicker approvals.
- Greater Transparency: Improved algorithms can provide clearer explanations for credit decisions, helping to build trust between lenders and borrowers.
Another trend includes the utilization of natural language processing (NLP). This technology will allow systems to analyze customer feedback and sentiments more effectively. Lenders can adjust their approaches based on real-time feedback from borrowers.
Moreover, as regulatory frameworks evolve, AI systems will likely need to align with new compliance standards. This necessity will drive innovation in developing tools that can swiftly adapt to regulatory changes while maintaining risk assessment accuracy.
Finally, ethical considerations will gain importance. Organizations must focus on ensuring their AI systems are free from bias and promote fairness in lending. Implementing guidelines for ethical AI use can enhance trust and foster long-term relationships with customers.
FAQ – Frequently Asked Questions about AI in Credit Risk Evaluation
How does AI improve credit risk assessments?
AI improves credit risk assessments by analyzing large data sets quickly and accurately, identifying patterns that human analysts may miss.
What are the benefits of using alternative data in credit evaluations?
Using alternative data helps provide a more comprehensive view of a borrower’s creditworthiness, leading to fairer and more informed lending decisions.
What challenges do financial institutions face when implementing AI?
Challenges include ensuring data quality, integrating AI with existing systems, and managing the costs of training staff and technology.
How can AI help build trust between lenders and borrowers?
AI can enhance transparency by providing clear explanations for credit decisions, which helps borrowers understand the evaluation process better.